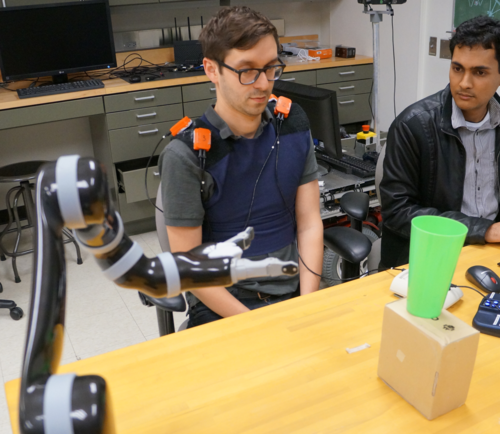
As assistive machines offer greater functionality, they often also become more complex to control. To operate such machines requires interfaces able to issue these complex signals, and the commercial interfaces which are available to those with severe motor impairments fall short—leaving those who arguably would benefit most from the assistive machine without access.
RIC’s novel Body Machine Interface (BoMI) has the potential to provide complex, high-dimensional control signals, by turning the body into a joysick (with the added benefit of promoting motor rehabilitation). The question we aim to answer in this project is whether this BoMI interface indeed can be used to control a complex assistive robot [13]?
Our proposed solution is to leverage robotics autonomy to handle a portion of the control, and gradually transfer more and more control to the human operator. To train the operator to generate higher and higher dimensional control signals, we additionally leverage machine learning in deciding when to unlock additional control dimensions for human operation.

In the first phase of this work, we performed a pilot study in which 10 uninjured participants operated the robotic arm via the BoMI over 5 sessions, without any autonomy-assisted learning paradigm. Our results revealed differences between joint-space and task-space control, in terms of both their initial intuition to operate and capacity for improvement over sessions [56, 58]. The next phase of this work involved the development of and vetting of the autonomy-assisted learning paradigm, which progressively unlocks control dimensions to the user, and also progressively scales back autonomy assistance. Our first step was to redesign the BoMI map to better meet the needs of our target end-user population, which included a scoping study with 3 SCI participants [61]. Next, 3 uninjured and 1 SCI participants vetted the autonomy-assisted learning protocol, which will soon launch as a 15-session study to be evaluated by SCI participants.
In collaboration with Lee Miller and Ferdinando Mussa-Ivaldi, Biomedical Engineering, Northwestern University and the Rehabilitation Institute of Chicago.
Funding Source: National Institutes of Health (R01-EB024058)