This project proposed a formalism for customizable shared control, that enables users to customize the way they share control with intelligent assistive devices based on their abilities and preferences. In our formalism, the system arbitrates between the user input and the autonomous policy prediction, based on the confidence it has in the policy’s prediction and in the user’s ability to perform the task.
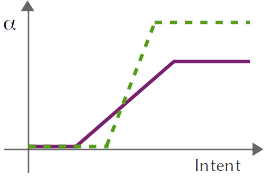
Our work on this project first focused on placing the customization of how control is shared directly in the hands of the end-user. An exploratory study with spinal cord injured and uninjured subjects allowed subjects to verbally customize the function which governed how control was shared [20].
We also customized intent inference to the end-user (a critical component of shared control), through a probabilistic interpretation of their control signals that allowed for suboptimality—for example, due to motor impairment or control interface limitations—through a metric that was custom-tuned to each human operator. Our study revealed that both the control interface in use and mechanism for intent inference indeed do impact shared-control operation, and that a customized probabilistic modeling of human actions results in faster assistance from the autonomy [31, 39].
Our work in autonomous grasp generation aimed to minimize any potential conflicts between the human and autonomy control signals during control sharing, by generating grasps that are semantically meaningful to humans and similar to how humans grasp objects. The algorithm furthermore does not rely on any object models, and thus is more flexibly deployed in real world Activities of Daily Living scenarios [16].
Funding Source: National Institutes of Health (NIH-R01EB019335)